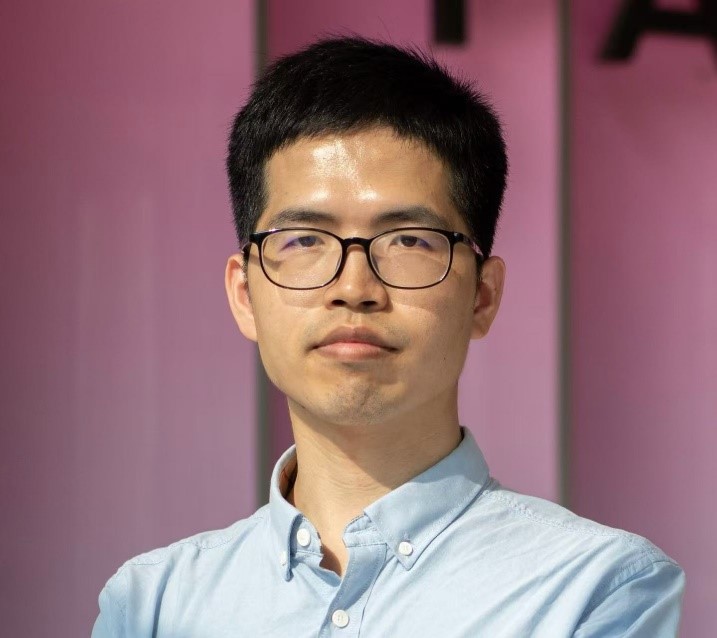
Prof. Yinyan Zhang, Jinan University, China
Biography: Yinyan Zhang is currently a Professor at Jinan University, Guangzhou, China. He received the B.E. degree in Automation from Sun Yat-sen University, Guanghzou, China, and the Ph.D. degree in Computing from The Hong Kong Polytechnic University, Hong Kong, China in 2016 and 2019, respectively. He worked as a Postdoctoral Research Fellow at Department of Computing in The Hong Kong Polytechnic University for a few months. Since Dec. 2019, he has been with the College of Cyber Security, Guangzhou, China. His research interests include dynamic neural networks, security and control of multi-agent systems, and robotics. He has published 32 papers in IEEE Transactions, such as IEEE TAC, IEEE TIE, IEEE TCST, IEEE TII, IEEE TNNLS, and IEEE TYCB, and 4 papers in Automatica. He is an Associate Editor of IEEE Transactions on Industrial Electronics and Neural Processing Letters. He is included in the World Top 2% Scientists ranking in 2021, 2022, 2023, and 2024.
Title: Dynamic Neural Networks for Multi-Agent Systems and Manipulator Control
Abstract: Dynamic neural networks originate from Hopfield neural networks named after John Joseph Hopfield, who has been awarded the 2024 Nobel Prize in physics for foundational discoveries and inventions that enable machine learning with artificial neural networks. Different from widely known forward neural networks, dynamic neural networks are often modeled with differential equations and rely on the core of control theory, i.e., feedback, and do not need offline training based on large amounts of data. In this talk, I shall discuss some works on dynamic neural networks for the distributed coordination and competition of multi-agent systems, especially distributed k-winners-take-all, which is useful for distributed task allocation in robotics. I shall also talk about some works on dynamic neural networks for manipulator control, especially kinematic control of redundant manipulators and visual servoing of manipulators.